Algorithms can improve equitable outcomes and can increase discrimination. How we evaluate the algorithms from conception to deployment will determine which way they go. I really liked two new papers this week addressing these two sides of the coin.
For algorithms that guide care, we need to rethink our validation processes: External validation of AI models in health should be replaced with recurring local validation (email me if you can’t access this). External validation assumes we can identify target populations and validate models on representative datasets before implementation.
Because models are deployed across healthcare facilities with vastly different operational, workflow and demographic characteristics, we need site-specific validation performed before every local deployment and repeated on a recurring basis. The clinical utility of models depends on how providers use model outputs. Provider actions and their interpretations of model outputs could differ across teams, facilities and over time.
Recurring local validation should also assess cost effectiveness, workflow disruptions, and fairness. If you know me, you have heard that the Validitron addresses part of this issue through using simulated settings to customize and validate digitally enabled workflows.
In Disparity dashboards: an evaluation of the literature and framework for health equity improvement, the authors point out that systems using algorithms to improve decision making can also exacerbate existing biases, including human bias, evidence bias, and those embedded in data trained on electronic health records.
A colleague of mine, Maia Hightower, created a company called Equality AI to help data scientists develop fair and unbiased algorithms to eliminate discrimination in machine learning models. Underlying their work is an Equality AI Manifesto that recognizes the fact that data scientists (and informaticists) are part of the healthcare team and therefore have specific responsibilities towards patients.
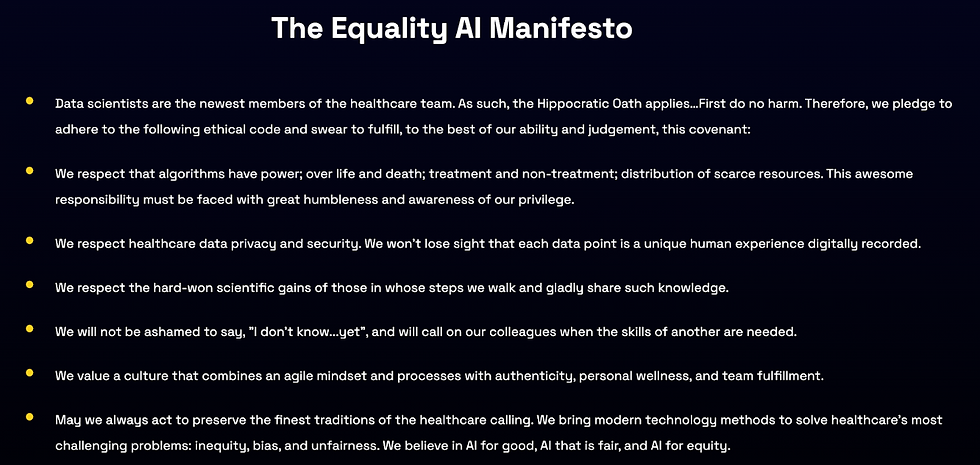
Algorithms can also help decrease disparities. Disparity dashboards can visualise data to highlight essential disparities in clinical outcomes and guide targeted quality improvement efforts.
Disparity dashboards build on the strengths of traditional clinical dashboards by not only identifying and monitoring disparities but also by helping to understand the underlying mechanism causing inequality. Creating useful dashboards will require careful mapping of data to variables that go beyond demographics to include underlying social or structural determinants of health and actionable information.
They developed this framework of important questions for developing clinically useful disparity dashboards that I look forward to exploring with our clinical partners.
![]() | ![]() |
Opmerkingen