Infrastructure: How can Academics Contribute to a Learning Health System? Part 2
- Wendy Chapman
- Jan 21, 2024
- 2 min read
"Why clinical AI is (almost) non-existent in Australian hospitals and how to fix it" is the name of a recent article by van der Vegt, Campbell, and Zuccon from University of Queensland. They say that in Australia, outside of digital imaging-based AI products, nearly all hospitals remain clinical AI-free zones. To illustrate this, they were only able to find two clinical AI stories in the country, and across what I assume is the Australian Alliance for AI in Healthcare only one hospital was known to have an AI trial underway.
The story is different elsewhere. You have probably heard about challenges with sepsis algorithms, but there has been progress, and eight sepsis prediction models have been implemented worldwide across 40 hospitals, all reporting reductions in mortality. There is still a stark contrast between the number of health AI publications--estimated to be 10,000/year--and the number of implemented AI applications.
Our Centre’s favourite analogy is between the drug development process, which has a widely agreed and clear pathway for innovation, and the digital health innovation process (figure by Daniel Capurro):
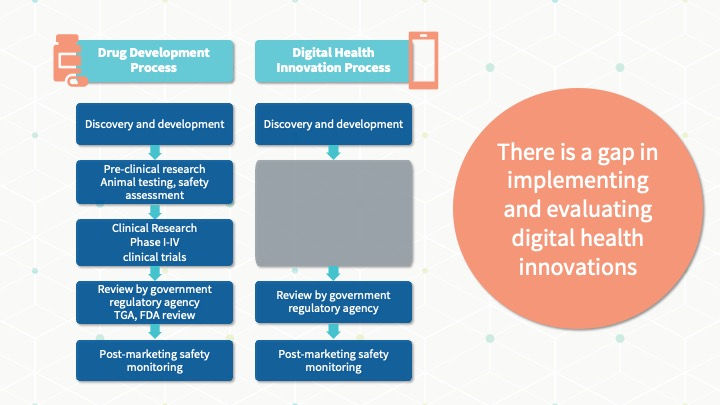
An important ingredient in addressing this gap is the academic LHS principle to capitalize on embedded academic expertise in health system sciences. Van der Vegt and colleagues synthesized 20 different frameworks to create the SALIENT end-to-end clinical AI implementation framework.

The first thing I see when I look at this figure is the need for layers of infrastructure to support AI developers in going from the problem definition phase to large scale rollout and generation of evidence. There are several resources being developed to address the diverse demands for implementation and adoption of AI and other technologies.
Standards and guidelines - The Coalition for Healthcare AI brings together industry, universities, government agencies, and healthcare organizations to provide guidelines for the responsible use of AI in healthcare. They focus on harmonizing standards and reporting for AI and education of end-users on how to evaluate these technologies to drive their adoption.
Supply Chain - The Mayo Clinic Platform AI Factory vertically integrates all parts of the AI supply chain into a single infrastructure, including data curation, data access and analytics, model validation, and production environment. This platform is driven by the belief that “even robustly built models using state-of-the-art algorithms may fail once tested in realistic environments due to unpredictability of real-world conditions, out-of-dataset scenarios, characteristics of deployment infrastructure, and lack of added value to clinical workflows relative to cost and potential clinical risks.”
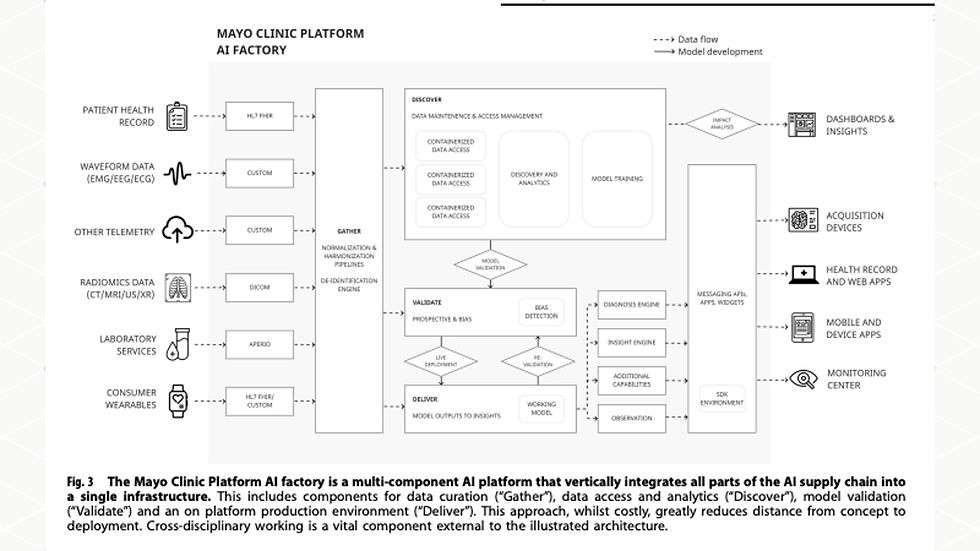
Implementability - At the University of Melbourne, we are developing the Digital Health Validitron with a focus on prototyping and validating digitally-enabled workflows. The SimLab is an immersive physical space designed to test and improve useability, acceptability, and safety of digital interventions. Workflow simulation is enabled by the digital sandbox that contains EMRs, telehealth tools, messaging systems, etc. so that you can replicate the IT ecosystem your innovation will be integrated into (slide courtesy Kit Huckvale).
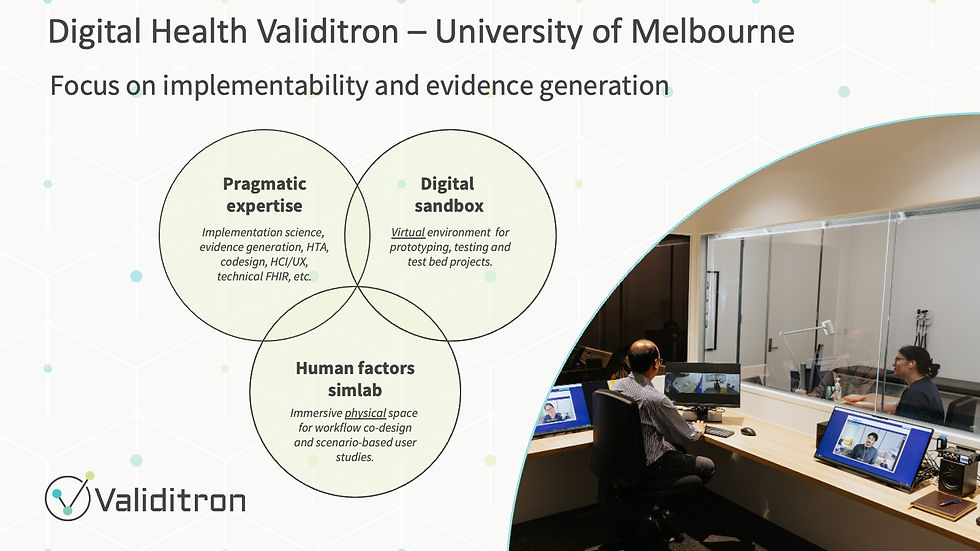
A common theme is the need to consider implementation and validation at the outset of technology design rather than treating it as an afterthought.
Another important observation from the SALIENT framework is the many disciplines that need to come to the table to translate AI and other innovations into healthcare, including researchers, regulators, clinicians, and managers/leaders. My next post on the academic LHS will focus on building effective partnerships when embedding academic expertise in health system sciences.
Very interesting insights on the slow adoption of clinical AI in Australian hospitals. It’s true that many healthcare systems are still hesitant to integrate AI beyond digital imaging. However, platforms like Doctiplus are leading the way in demonstrating how AI can be used effectively in telehealth to improve patient care and streamline processes. Academic contributions are crucial in overcoming these barriers by creating the infrastructure needed for AI to be safely and effectively implemented across clinical settings. Hopefully, with more collaboration between academia and healthcare providers, we can see greater AI integration in the future.