Let's talk about AI
- Wendy Chapman
- Aug 13, 2023
- 2 min read
In particular, generative AI using large language models. They are on everyone’s mind, and papers and talks abound to help us think about their application in healthcare. I want to share a talk and a paper from last week that I found thought provoking.
Brian found an insightful talk by Pete Szolovits at a symposium called The Impact of chatGPT (on YouTube). Pete leads the CSAIL Clinical Decision Making Group at MIT and has been applying computational models to health and decision making since the 1970’s--in fact, he inspired my mentor Greg Cooper as an undergraduate to go into medical applications of computer science.
In the talk, Pete states the stunning truth that nobody actually knows how and why ChatGPT works. He and his group asked the question “Do we still need clinical language models?” They tested the T5 model on three clinical tasks using different sizes of training data (text) and conclude that more training data is better, but in-domain data is better yet. And, of course, the combination performs the best.
He concludes by saying that “Generative AI seems miraculous…but science abhors miracles.” Most current research focuses on how to use generative AI models to improve applications, but there is hardly any research on why these capabilities work in the first place. Is it safe to focus on applying them in healthcare before we even understand how they work?
A recent article in JAMA Network (by my colleague Nigam Shah and David Entwistle, CEO of Stanford health who was the CEO at University of Utah Health when I started as the chair) calls for more clinician involvement in large language models applied to healthcare.
The medical profession has made a mistake in not shaping the creation, design, and adoption of most information technology systems in health care. Given the profound disruption that is possible for such diverse activities… the same mistake cannot be repeated.
They suggest two foundational questions we should be asking:
1. Are the LLMs Being Trained With the Relevant Data and the Right Kind of Self-Supervision?
[H]ealth care systems should be training shared, open-source models using their own data. The technology companies should be asked whether the models being offered have seen any medical data during training and whether the nature of self-supervision used is relevant for the final use of the model.
Are our Australian hospitals thinking in this way?
2. Are the Purported Value Propositions of Using LLMs in Medicine Being Verified?
The purported benefits need to be defined and evaluations conducted to verify such benefits
Of course, we believe the Validitron could be one way to validate the purported benefits of AI before rolling the algorithms out into real health settings, but there is a lot of thinking and experimenting to be done to know how to do this well.
They conclude their article with an instructive figure and this warning:
By not asking how the intended medical use can shape the training of LLMs and the chatbots or other applications they power, technology companies are deciding what is right for medicine.
Tuesday, I am lucky to be participating in the National AI in Healthcare Policy Workshop in Sydney hosted by the Australian Alliance for AI in Healthcare and will report back!
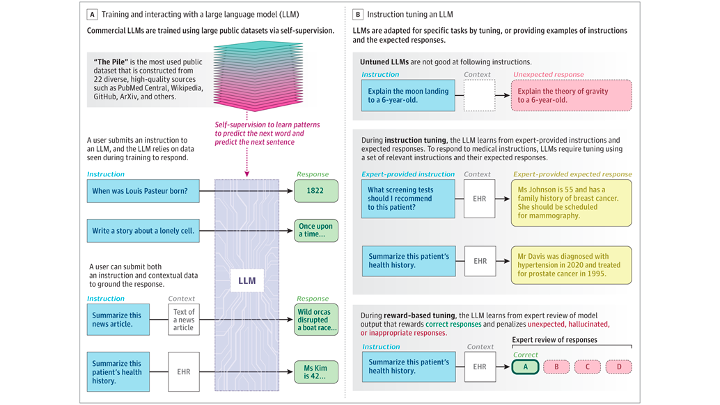
Great post, quick thought on Pete's talk -
We use many medications where the mechanism of action (how it works) is unknown or theoretical (e.g., Panadol). They have been found to be safe and effective in all phase trials so they are used. Since the FDA does not require the intervention to prove how it works, why is it important for LLM? Should we instead prioritise other questions? Of course, knowing how something works is nice to have but is it crucial?